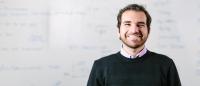
Cardiology Fellow Pierre Elias Named STAT Wunderkind
Pierre Elias, MD, a cardiology fellow at the Columbia University Vagelos College of Physicians and Surgeons and NewYork-Presbyterian, has been named a 2020 STAT Wunderkind. STAT is a leading publication in medical news, and its annual Wunderkind list honors early career physicians and scientists who are doing groundbreaking work in their field.
Before medical school, Elias worked for a startup whose technology was acquired by Google. As a cardiology fellow, he is applying his informatics knowledge to a field where it could save lives.
Elias is researching the use of machine learning to improve care for patients with heart disease. Finding patterns within patients’ ECG data could allow doctors to get earlier warnings of problems that often lead to heart failure and death. This year he worked with Madiha Choksi, a research technologist at the Columbia University Libraries, to rapidly produce face shields with 3D printing to reduce shortages of PPE due to the coronavirus pandemic.
We spoke to Elias about his work and unique background.
Why did you move from tech to cardiology?
I feel that the tech world can help you develop a toolset to create change at a large scale, but medicine is where I found the problems and purpose that mattered to me. I’m most fulfilled at the intersection of two, where I can see patients one at a time but build systems that help all clinicians deliver their version of ideal care every time.
What are you working on now, and how can it change health care?
We know that there are lots of patients out there with undetected cardiac diseases, like aortic stenosis, where one of the valves on your heart is too tight. These patients can develop severe disease before it's finally picked up, but the sooner we know about it the better we can take care of them.
So what we do is we take a very cheap and commonly done test—a 12-lead electrocardiogram (ECG)—and we train a deep learning model to find aortic stenosis on the ECG. We provide the model with data from patients who have had both an ECG and the more expensive diagnostic test for aortic stenosis (an ultrasound of the heart called an echocardiogram).
As a cardiologist I can’t tell if a patient has aortic stenosis by their ECG, but it turns out these deep learning models can do exactly that.
The goal is to develop a system that automatically flags patients that are at high risk for certain cardiac diseases as soon as they have an ECG done, before they have any symptoms.
What's the best thing about working at Columbia?
Without a doubt it’s the people. My collaborators and mentors are people who have helped me develop this work from day one. This is truly a team effort and my co-captain in this work, Tim Poterucha [a postdoctoral clinical fellow at Columbia], is not only an incredible researcher but also a great friend. Getting to love what you do and who you work with is rare, and I’m grateful to have found that at Columbia.